DSE Capstone - American Gut Project Cohort4 2019
Project Readme
File Size |
|
File Format |
|
Scope And Content | Information about the data, main github repo, and visualization repo |
Main DSE GitHub repository
File Size |
|
File Format |
|
Scope And Content | Various analysis scripts for project and a final american_gut_project library that includes a pipeline workflow |
Technical Details |
Pipeline pip packages: |
DSE AGP visualization repo
File Size |
|
File Format |
|
Scope And Content | Visualization dashboard for project |
Raw & Output data
File Size |
|
File Format |
|
Scope And Content | README and git repo contains information on the raw and output data |
License File
File Size |
|
File Format |
|
Scope And Content | License information |
Final Poster
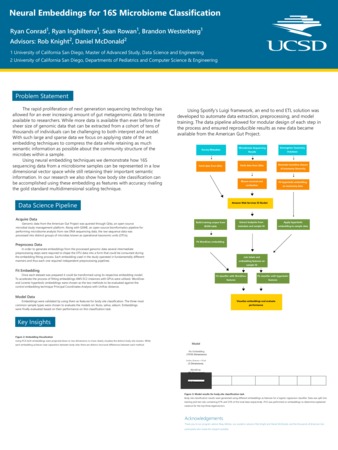
File Size |
|
File Format |
|
Scope And Content | Poster from final submission |
- Collection
- Cite This Work
-
Conrad, Ryan; Inghilterra, Ryan; Rowan, Sean; Westerberg, Brandon; McDonald, Daniel; Knight, Rob (2019). DSE Capstone - American Gut Project Cohort4 2019. In Data Science & Engineering Master of Advanced Study (DSE MAS) Capstone Projects. UC San Diego Library Digital Collections. https://doi.org/10.6075/J0HT2MN3
- Description
-
Abstract:
The American Gut Project (AGP) [1] is the largest citizen crowd-sourced collection of gut microbiome samples available today. Knowledge of the microbiome is in its beginning stages and the enormous amount of organism and gene effects that are ill-understood makes accurately interpreting results difficult. Reducing this high dimensional space with fundamentally different embedding techniques can be effective in capturing different aspects of the microbiome data to aide in research. Dimensionality reduction techniques like Word2Vec, Hyperbolic Embeddings, and Principal Coordinates Analysis (PCoA) were used to reduce a single sample’s dimensionality and explore their different strengths. Embeddings were validated by using them as features for a supervised machine learning model that classifies microbiome body sites (e.g. sebum, feces, saliva). Competing against the state of the art of PCoA using underlying phylogeny distances, the different embeddings kept the baseline logistic regression model’s F1 score within acceptable margins at +/- 0.1. These reduction comparisons included actual dimension sizes, metrics of the model prediction, and a representation of samples’ clusters. This paper will discuss the analysis, architecture, and visualization of the project that approached this main technical challenge of gaining a better understanding of microbiota.
This project was done in the Cohort 4 2017-2019 group for the MAS DSE Master's program. The data used comes from the Rob Knight UCSD Lab and is contained in the Qiita website under study #10317.
This project contains various analyses on microbiome data, survey data, drug data, and diet data. It also contains a Luigi pipeline and a Plotly Dash application for front end usage. - Creation Date
- 2019-01-04 to 2019-06-06
- Date Issued
- 2019
- Authors
- Advisors
- Series
- Topics
Formats
View formats within this collection
- Language
- English
- Identifier
- Related Resources
- Qiita Study Data 10317: https://qiita.ucsd.edu/study/description/10317
- Github Group 1 Vis repo: https://github.com/mas-dse-ringhilt/american_gut_capstone_dashboard
- Github Group1 repository: https://github.com/mas-dse-ringhilt/DSE-American-Gut-Project
- Knight Lab AGP repo: https://github.com/knightlab-analyses/american-gut-analyses/tree/master/ipynb
- DrugBank 5.0: a major update to the DrugBank database for 2018. Wishart DS, Feunang YD, Guo AC, Lo EJ, Marcu A, Grant JR, Sajed T, Johnson D, Li C, Sayeeda Z, Assempour N, Iynkkaran I, Liu Y, Maciejewski A, Gale N, Wilson A, Chin L, Cummings R, Le D, Pon A, Knox C, Wilson M. Nucleic Acids Res. 2017 Nov 8. https://doi.org/10.1093/nar/gkx1037
- McDonald D, Hyde E, Debelius JW, et al. 2018. American Gut: an open platform for citizen science microbiome research. mSystems 3:e00031-18. https://doi.org/10.1128/mSystems.00031-18
- Qiita: rapid, web-enabled microbiome meta-analysis, (Specifically Study ID 10317 Used) Antonio Gonzalez, Jose A. Navas-Molina, Tomasz Kosciolek, Daniel McDonald, Yoshiki Vázquez-Baeza, Gail Ackermann, Jeff DeReus, Stefan Janssen, Austin D. Swafford, Stephanie B. Orchanian, Jon G. Sanders, Joshua Shorenstein, Hannes Holste, Semar Petrus, Adam Robbins-Pianka, Colin J. Brislawn, Mingxun Wang, Jai Ram Rideout, Evan Bolyen, Matthew Dillon, J. Gregory Caporaso, Pieter C. Dorrestein & Rob Knight. Nature Methods, volume 15, pages 796–798 (2018). https://doi.org/10.1038/s41592-018-0141-9
Source data
Software
Reference
- License
-
Creative Commons Attribution 4.0 International Public License
- Rights Holder
- Conrad, Ryan; Inghilterra, Ryan; Rowan, Sean; Westerberg, Brandon; McDonald, Daniel; Knight, Rob
- Copyright
-
Under copyright (US)
Use: This work is available from the UC San Diego Library. This digital copy of the work is intended to support research, teaching, and private study.
Constraint(s) on Use: This work is protected by the U.S. Copyright Law (Title 17, U.S.C.). Use of this work beyond that allowed by "fair use" or any license applied to this work requires written permission of the copyright holder(s). Responsibility for obtaining permissions and any use and distribution of this work rests exclusively with the user and not the UC San Diego Library. Inquiries can be made to the UC San Diego Library program having custody of the work.
- Digital Object Made Available By
-
Research Data Curation Program, UC San Diego, La Jolla, 92093-0175 (https://lib.ucsd.edu/rdcp)
- Last Modified
2023-06-06