LLNL D4DCT Datasets: Dynamic 4DCT Datasets using MPM-based Deformation, 80^3 volume resolution
Additional dataset description (LLNL_D4DCT_Datasets.pdf)
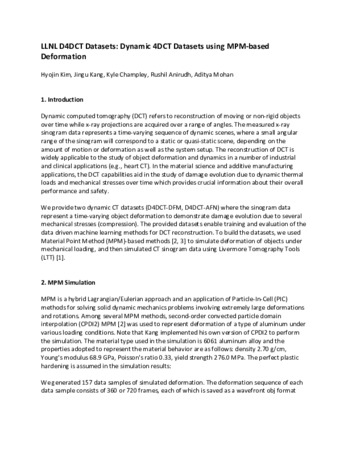
File Size |
|
File Format |
|
Description | Describes MPM-based deformation, CT simulation setup, and data categories. |
Dynamic 4DCT Dataset of MPM-based Deformation (D4DCT-DFM)
File Size |
|
File Format |
|
Description | Contains 157 different deformation sets, each of which has 20 different data samples. |
Dynamic 4DCT Dataset of Affine Transformed Objects (D4DCT-AFN)
File Size |
|
File Format |
|
Description | Contains 157 different deformation sets, each of which has 20 different data samples. |
- Collection
- Cite This Work
-
Kim, Hyojin; Kang, Jingu; Champley, Kyle; Anirudh, Rushil; Mohan, Aditya (2020). LLNL D4DCT Datasets: Dynamic 4DCT Datasets using MPM-based Deformation, 80^3 volume resolution. In Lawrence Livermore National Laboratory (LLNL) Open Data Initiative. UC San Diego Library Digital Collections. https://doi.org/10.6075/J00R9MZF
- Description
-
Dynamic computed tomography (DCT) refers to reconstruction of moving or non-rigid objects over time while x-ray projections are acquired over a range of angles. The measured x-ray sinogram data represents a time-varying sequence of dynamic scenes, where a small angular range of the sinogram will correspond to a static or quasi-static scene, depending on the amount of motion or deformation as well as the system setup. The reconstruction of DCT is widely applicable to the study of object deformation and dynamics in a number of industrial and clinical applications (e.g., heart CT). In the material science and additive manufacturing applications, the DCT capabilities aid in the study of damage evolution due to dynamic thermal loads and mechanical stresses over time which provides crucial information about their overall performance and safety.
We provide two dynamic CT datasets (D4DCT-DFM, D4DCT-AFN) where the sinogram data represent a time-varying object deformation to demonstrate damage evolution due to several mechanical stresses (compression). The provided datasets enable training and evaluation of the data driven machine learning methods for DCT reconstruction. To build the datasets, we used Material Point Method (MPM)-based methods to simulate deformation of objects under mechanical loading, and then simulated CT sinogram data using Livermore Tomography Tools (LTT). - Creation Date
- 2020-05-10 to 2020-10-30
- Date Issued
- 2020
- Principal Investigator
- Co Principal Investigators
- Data Contributors
- Funding
-
Laboratory Directed Research and Development (LDRD)-FS: 20-FS-010
- Topics
Formats
View formats within this collection
- Language
- English
- Identifier
-
Identifier: Aditya Mohan: http://orcid.org/0000-0002-0921-6559
Identifier: Hyojin Kim: https://orcid.org/0000-0001-7032-0999
Identifier: Rushil Anirudh: https://orcid.org/0000-0002-4186-3502
- Related Resources
- Albert W. Reed, Hyojin Kim, Rushil Anirudh, K. Aditya Mohan, Kyle Champley, Jingu Kang, Suren Jayasuriya; Dynamic CT Reconstruction From Limited Views With Implicit Neural Representations and Parametric Motion Fields. Proceedings of the IEEE/CVF International Conference on Computer Vision (ICCV), 2021. https://openaccess.thecvf.com/content/ICCV2021/html/Reed_Dynamic_CT_Reconstruction_From_Limited_Views_With_Implicit_Neural_Representations_ICCV_2021_paper.html
- Albert W. Reed, Hyojin Kim, Rushil Anirudh, K. Aditya Mohan, Kyle Champley, Jingu Kang, Suren Jayasuriya (2021). Dynamic CT Reconstruction from Limited Views with Implicit Neural Representations and Parametric Motion Fields. arXiv. https://arxiv.org/abs/2104.11745
- Dynamic CT Reconstruction from Limtied Views with Implicit Neural Representations and Parametric Motion Fields GitHub repository: https://github.com/awreed/DynamicCTReconstruction
- Kim, Hyojin; Kang, Jingu; Champley, Kyle; Anirudh, Rushil; Mohan, Aditya (2022). LLNL D4DCT Datasets: Dynamic 4DCT Datasets using MPM-based Deformation, 256^3 volume resolution. In Lawrence Livermore National Laboratory (LLNL) Open Data Initiative. UC San Diego Library Digital Collections. https://doi.org/10.6075/J0765FG7
Primary associated publication
Software
Related data
- License
-
Creative Commons Attribution 4.0 International Public License
- Rights Holder
- Lawrence Livermore National Laboratory
- Copyright
-
Under copyright (US)
Use: This work is available from the UC San Diego Library. This digital copy of the work is intended to support research, teaching, and private study.
Constraint(s) on Use: This work is protected by the U.S. Copyright Law (Title 17, U.S.C.). Use of this work beyond that allowed by "fair use" or any license applied to this work requires written permission of the copyright holder(s). Responsibility for obtaining permissions and any use and distribution of this work rests exclusively with the user and not the UC San Diego Library. Inquiries can be made to the UC San Diego Library program having custody of the work.
- Digital Object Made Available By
-
Research Data Curation Program, UC San Diego, La Jolla, 92093-0175 (https://lib.ucsd.edu/rdcp)
- Last Modified
2024-05-01